This software allows users to input seven variables, such as Tmin, Tmax, and WSPD, and run the KNN-WG algorithm using their data.
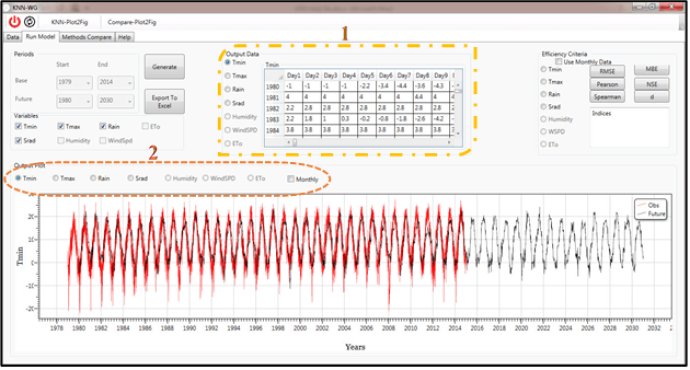
One of the most popular applications of K-NN is the generation of weather data. It is based on recognizing a similar pattern of target data within the historical observed weather data that could be used to reduce the target year. K-NN relies on the assumption that the actual weather data observed during the target year could be a replication of weather recorded in the past. The target year and historical data are required as input files to run the model.
K-NN technique does not use any predefined mathematical functions to estimate a target variable. In fact, the algorithm of this method typically involves selecting a specified number of days similar in characteristics to the day of interest. One of these days is randomly resampled to represent the weather of the next day in the simulation period. The nearest neighbor approach involves simultaneous sampling of the weather variables, such as precipitation and temperature, from the observed data with replacement.
The K-NN method is widely used in agriculture, forestry, and hydrology. It has proven to be an effective and reliable technique for generating weather data. Several researchers including Young, Yates, Eum, Bannayan, Hoogenboom, Lopez, Clark, and Yates have highlighted the importance of K-NN in their studies. Overall, the K-nearest neighbor (K-NN) method is an excellent approach for generating weather data and should be considered when conducting future studies.
Version 1.0: N/A